DIONE In-situ component
Foresees to establish an ecosystem of low-cost smart tools to complement EO based monitoring. It includes a geotagged photos framework (consisting of mobile application for data collection and a backend part for integrity/anonymization of received data), portable soil spectrometers working in tandem with mobile application, a backend tool to support pre-processing, validation and management of soil spectra received, machine learning models to convert initially soil spectra to soil properties (point observations) and then the latter to maps covering larger areas.
1. Geotagged photos framework
DIONE farmers’ geotagged photos framework comprises different components and technical innovations towards assisting and guiding users to capture efficiently representative photos of their parcels while adhering to current technical recommendations and ensuring the security, validity and reliability of the collected photos.
The data collection process is supported by a mobile application that allows users to view related content about their parcels, receive notifications about tasks that they need to undertake as well as get Augmented Reality enabled directions regarding the process of capturing appropriately photos of a given parcel.
Additionally, in order to ensure that the file of the photo captured by the mobile application is not manipulated and is the same with respect to the location and time the photo was taken, an integrity framework has been also employed. Different algorithms have been implemented for a multifaceted solution and better security.
The application is accessible on Play Store
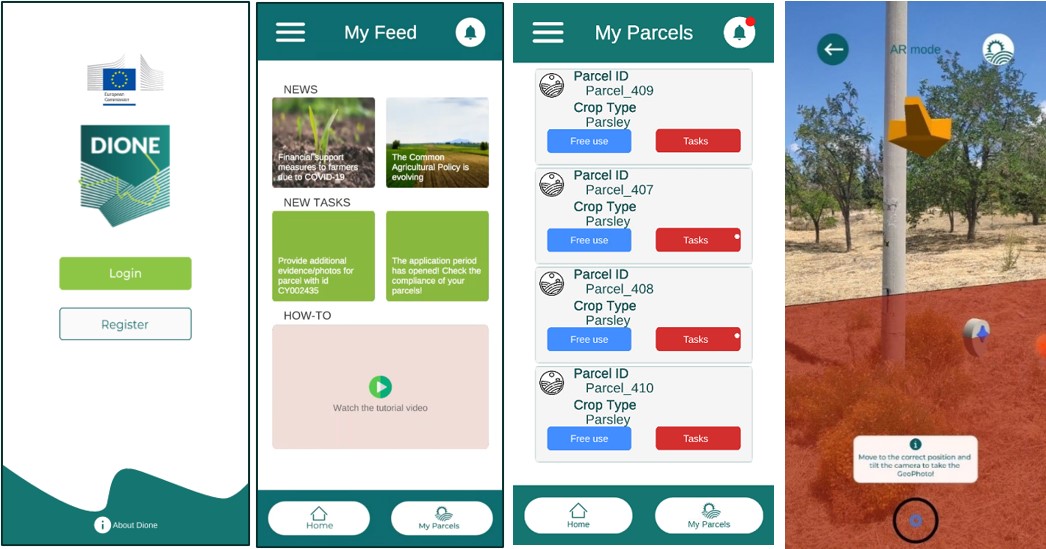
Geotagged photos
2. In-situ soil scanning system
DIONE’s soil scanning system is based on a suitably selected low-cost spectral sensor employing microelectromechanical systems (MEMS) technology. This sensor records the diffuse reflectance spectrum in the near infrared (1750 to 2150 nm) which is affected by several key soil properties. The spectrometer is operated through an Android application via a Bluetooth connection. In addition to the soil spectrum, several metadata are recorded including the timestamp, latitude and longitude of the sample, its humidity level, and more.
The data are automatically transmitted to a central database for storage. Furthermore, at this stage a set of data pre-processing (including spectral standardization), outlier and novelty detection techniques are automatically applied to ensure the integrity and quality of the collected measurements.
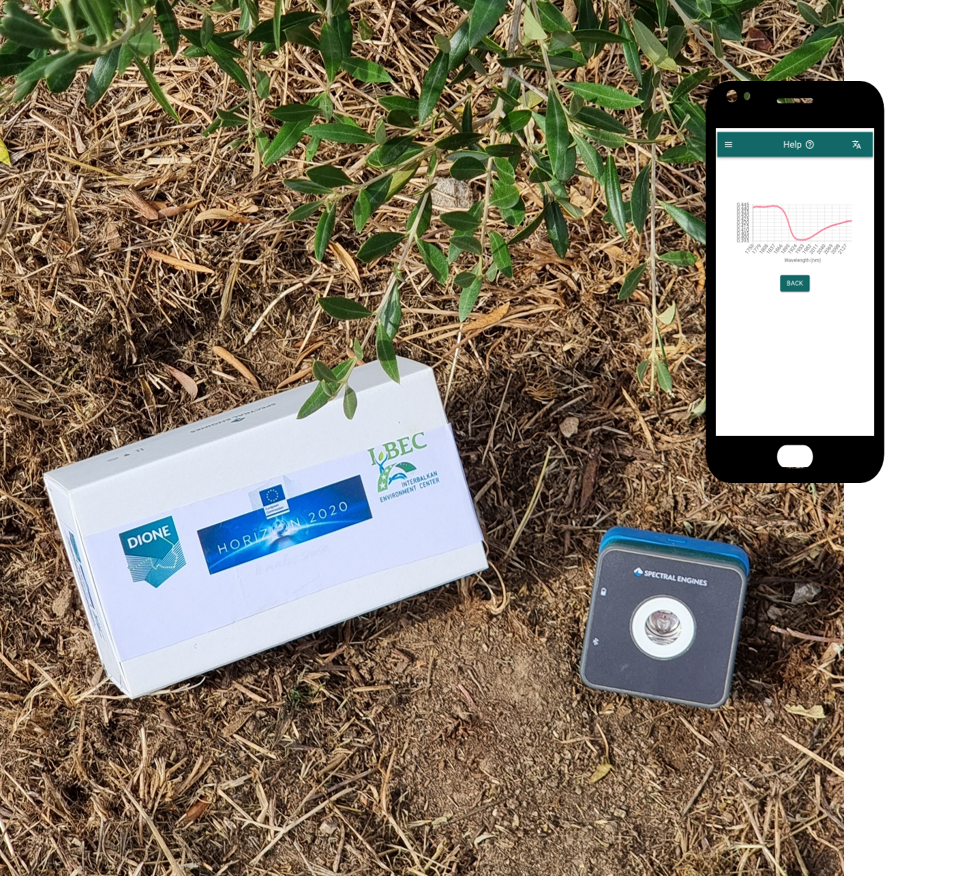
Soil Scanning System
3. ML based algorithms for the extraction of soil properties and soil degradation maps
It covers the development of novel machine learning tools which will transform the raw data collected through the in-situ soil scanning system to the appropriate soil properties (SOC, clay, pH and CaCO3) as well as a second toolset of machine learning algorithms which will appropriately combine these point measurements with EO imagery and produce maps for the areas of interest.
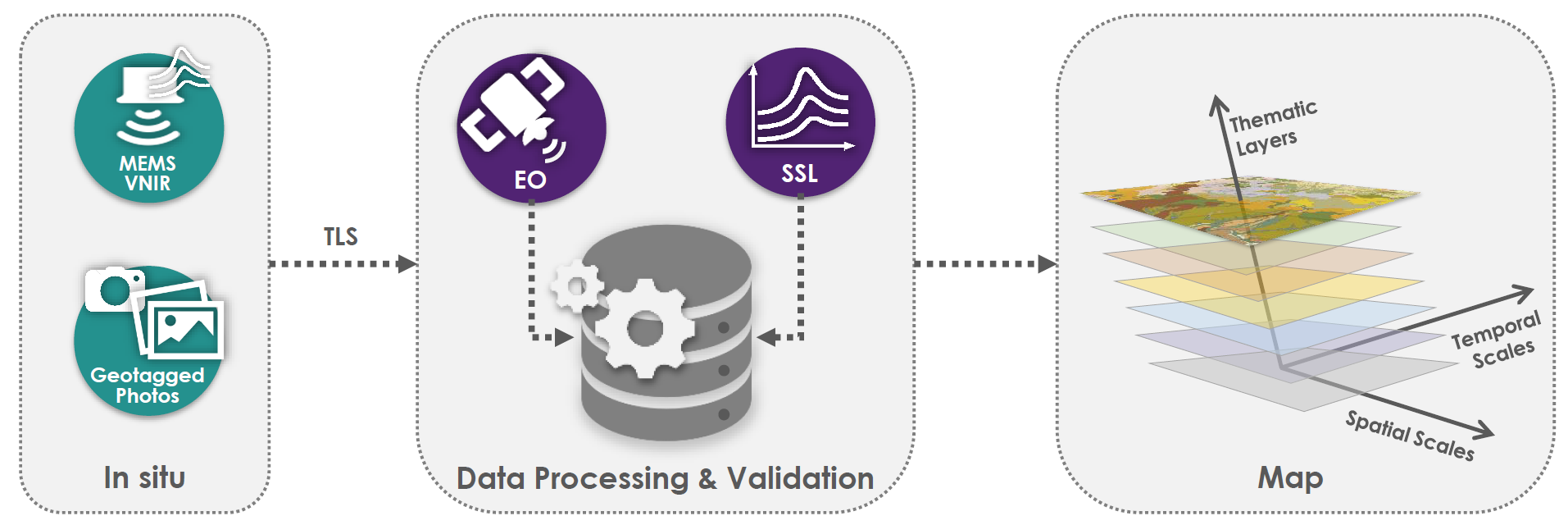
Machine Learning (ML) Based Algorithms